A “moat” historically meant a physical method to reduce threats to a group intended to fit inside it. Take for example the large Buhen fortress on the banks of the Nile. Built by Pharaoh Senwosret III around 1860 BCE, it boasted a high-tech ten meter high wall next to a three meter deep moat to protect his markets against Nubians who were brave enough to fight against occupation and exploitation.
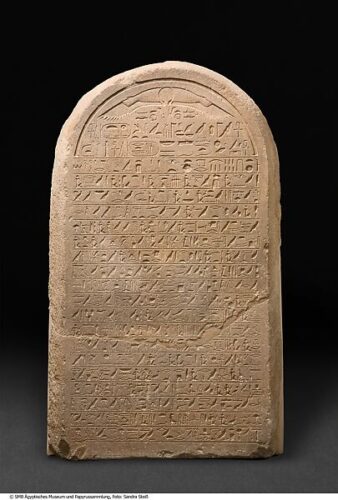
Egyptian Boundary Stele of Senwosret III, ca. 1878-1840 B.C., Middle Kingdom. Quartzite; H. 160 cm; W. 96 cm. On loan to Metropolitan Museum of Art, New York (MK.005). http://www.metmuseum.org/Collections/search-the-collections/591230
Complicated, I suppose, since being safe inside such a moat meant protection against threats, yet being outside was defined as being a threat.
Go inside and lose freedom, go outside and lose even more? Sounds like Facebook’s profit model can be traced back to stone tablets.
Anyway, in true Silicon Valley fashion of ignoring complex human science, technology companies have been expecting to survive an inherent inability to scale by relying on building primitive “moats” to prevent groups inside from escaping to more freedom.
Basically moats used to be defined as physically protecting markets from raids, and lately have been redefined as protecting online raiders from markets. “Digital moats” are framed for investors as a means to undermine market safety — profit from users enticed inside who then are denied any real option to exit outside.
Unregulated highly-centralized proprietary technology brands have modeled themselves as a rise of unrepresentative digital Pharoahs who are shamelessly attempting new forms of indentured servitude despite everything in history saying BAD IDEA, VERY BAD.
Now for some breaking news:
Google has been exposed by an alleged internal panic memo about profitability of future servitude, admitting “We Have No Moat, And Neither Does OpenAI”
While we’ve been squabbling, a third faction has been quietly eating our lunch. I’m talking, of course, about open source. Plainly put, they are lapping us. Things we consider “major open problems” are solved and in people’s hands today. […] Open-source models are faster, more customizable, more private, and pound-for-pound more capable. They are doing things with $100 and 13B params that we struggle with at $10M and 540B. And they are doing so in weeks, not months.
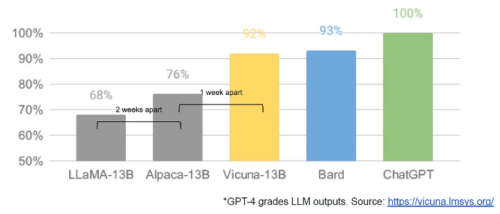
It’s absolutely clear this worry and fret from Google insiders comes down to several key issues. The following paragraph in particular caught my attention since it feels like I’ve been harping about this for at least a decade already:
Data quality scales better than data size
Many of these projects are saving time by training on small, highly curated datasets. This suggests there is some flexibility in data scaling laws. The existence of such datasets follows from the line of thinking in Data Doesn’t Do What You Think, and they are rapidly becoming the standard way to do training outside Google.
There has to be common sense about this. Anyone who thinks about thinking (let alone writing code) knows a minor change is more sustainable for scale than complete restarts. The final analysis is that learning improvements grow bigger faster and better through fine-tuning/stacking on low-cost consumer machines instead of completely rebuilding upon each change using giant industrial engines.
…the model can be cheaply kept up to date, without ever having to pay the cost of a full run.
You can scale a market of ideas better through a system designed for distributed linked knowledge with safety mechanisms, rather than planning to build a new castle wall every time a stall is changed or new one opened.
Building centralized “data lakes” was a hot profit ticket in 2012 that blew-up spectacularly just a few years later. I don’t think people realized social science theory like “fog of war” had told them not to do it, but they definitely should have walked away from “largest” thinking right then.
Instead?
OpenAI was born in 2015 on the sunset phase of a wrong model mindset. Fun fact: I once was approached and asked to be CISO for OpenAI. Guess why I immediately refused and instead went to work on massively distributed high-integrity models of data for AI (e.g. W3C Solid)?
…maintaining some of the largest models on the planet actually puts us at a disadvantage.
Yup. Basically confidentiality failures that California breach law SB1386 hinted at way back in 2003, let alone more recent attempts to stop integrity failures.
Tech giants have vowed many times to combat propaganda around elections, fake news about the COVID-19 vaccines, pornography and child exploitation, and hateful messaging targeting ethnic groups. But they have been unsuccessful, research and news events show.
Bad Pharaohs.
Can’t trust them, as the philosopher David Hume sagely warned in the 1700s.
To me the Google memo reads as if pulled out of a dusty folder: an old IBM fret that open communities running on Sun Microsystems (get it? a MICRO system) using wide-area networks to keep knowledge cheaply up to date… will be a problem for mainframe profitability that depends on monopoly-like exit barriers.
Exiting times, in other words, to be working with open source and standards to set people free. Oops, meant to say exciting times.
Same as it’s ever been.
There is often an assumption that operations should be large and centralized in order to be scalable, even though such thinking is provably backwards.
I suspect many try to justify such centralization due to cognitive bias, not to mention hedging benefits away from a community and into a just small number of hands.
People sooth fears through promotion of competition-driven reductions; simple “quick win” models (primarily helping themselves) are hitched to a stated need for defense, without transparency. They don’t expend effort on wiser, longer-term yet sustainable efforts of more interoperable, diverse and complex models that could account for wider benefits.
The latter models actually scale, while the former models give an impression of scale until they can’t.
What the former models do when struggling to scale is something perhaps right out of ancient history. Like what happens when a market outgrows the slowly-built stone walls of a “protective” monarchist’s control.
Pharoahs are history for a reason.