Back in September 2014 there was an excellent article on FP called “Why Big Data Missed Early Warning Signs of Ebola“, which seems more relevant today than ever:
It’s an inspirational story that is a common refrain in the big data world — sophisticated computer algorithms sift through millions of data points and divine hidden patterns indicating a previously unrecognized outbreak that was then used to alert unsuspecting health authorities and government officials. The problem is that this story isn’t quite true: By the time HealthMap monitored its very first report, the Guinean government had actually already announced the outbreak and notified the WHO.
The FP article goes on to clarify the problem was never a lack of social commentary to monitor, which legitimately came early and wasn’t even noticed by big data systems anyway. The problem was that official channels of news were downplayed by purveyors of “artificial intelligence” (AI) to take all the credit by simply repeating those very same official channels of news.
Thus, contrary to the narrative that data mining led to an intelligence coup, HealthMap’s earliest signals on March 14 were actually simply detections of this official government announcement in French. Despite all of the attention and hype paid to social media as a sensor network over human society, mainstream media still plays a critical role as an information stream in many areas of the world. This is not to say that there were not far earlier signals manifested in the myriad social conversations among medical workers and citizens in the region, only that it was not these indicators that HealthMap — or anyone else — detected.
My presentations in 2014 and after would often cite this example as a failure of big data, as well as the Google flu prediction engine crashing and burning from integrity failures.
Most recently at the 2019 RSA Conference for example, I presented this story of failed Ebola warnings as one of the top ten security disasters of ML.
My presentations since 2014 also have included references to insurance companies running very secretive big data systems in the cloud to model pandemics — not to mention chemical weapons — spreading in America (since at least 2008 federal security researchers have said a pandemic is a greater threat to the US than nuclear attack). Did you know the insurance rates of a commercial property may have several pandemic models in its estimated risk? Perhaps I will dig up some of my old 2014 slides and post here again to illustrate better.
One true “in the trenches of big data technology” experience I used to like to present, for example, was how one very large insurance company got a phone call from Amazon demanding some kind of formal advance notice before its cloud services were lit up for pandemic simulations. 2014 was a time when the whole of Amazon’s cloud simply couldn’t handle the loads of powerful and real pandemic prediction models based on truly big data.
A lot has changed since then, although some things have not. Let’s talk now about COVID-19.
On the plus side a pandemic-prediction technology company founded during the Ebola crisis has recently claimed success in the early warning game:
…December 30, 2019, BlueDot, a Toronto-based startup that uses a platform built around artificial intelligence, machine learning and big data to track and predict the outbreak and spread of infectious diseases, alerted its private sector and government clients about a cluster of “unusual pneumonia” cases happening around a market in Wuhan, China. That was the first recognition of the novel coronavirus that has come to be known as COVID-19.
Before looking at this tall claim more carefully, note the list of “first places” in the same story:
In the case of COVID-19, the system flagged articles in Chinese that reported 27 pneumonia cases associated with a market that had seafood and live animals in Wuhan. In addition to the alert, BlueDot correctly identified the cities that were highly connected to Wuhan using things like global airline ticketing data to help anticipate where the infected might be traveling. The international destinations that BlueDot anticipated would have the highest volume of travelers from Wuhan were: Bangkok, Hong Kong, Tokyo, Taipei, Phuket, Seoul, and Singapore. In the end, 11 of the cities at the top of their list were the first places to see COVID-19 cases.
Here they are again:
- Bangkok in Thailand
- Hong Kong
- Tokyo in Japan
- Taipei in Taiwan
- Phuket in Thailand
- Seoul in South Korea
- Singapore
In reality the initial confirmed spread outside China went to the US as well as Taiwan, Thailand, Japan and South Korea.
Now look at the lines on the following Tomas Pueyo graph of infection rates from his post called “Act Today or People Will Die“.
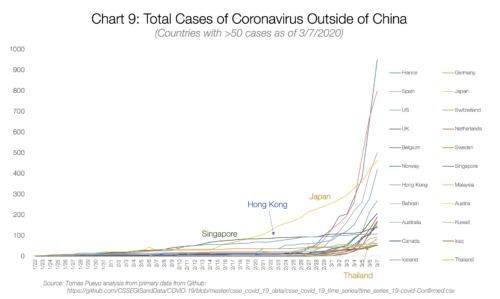
If you squint you may be able to see the cities listed at the top of the BlueDot list are near to flat on the bottom of the chart, unless they’re not on the chart at all because too few cases exist.
Countries like South Korea, US and France are rocketing upwards. As the author explains without mincing words, there’s an obvious causation for the difference in rates:
South Korea cases have exploded, but have you wondered why Japan, Taiwan, Singapore, Thailand or Hong Kong haven’t? All of them were hit by SARS in 2003, and all of them learned from it.
SARS had a huge impact in 37 countries. The ones that setup national pandemic command centers, getting prepared for the next virus, are showing direct benefits. Their use of big data has been to enhance preparedness by enabling testing and containment routines, best exemplified by the Singapore public dashboard.
Meanwhile in America, the lessons from the spread of a deadly virus seem to have been mostly ignored or reversed by the current administration, leading the country towards a repeat of tragic American history.
It’s time we go back to 1981 when American scientists initially noticed a new virus because unusual levels of the uncommon drug Pentamidine were being prescribed. That kind of uptick in consumption is a text-book early warning sign for big data systems to easily understand.
However it took another five long years under President Ronald Reagan before there was even a statement made about deaths from human immunodeficiency virus (HIV) that had been flagged in 1981. FIVE YEARS and 25,000 dead Americans happened before the President started to focus on HIV. There was open ignorance and dismissal from 1982 to 1987 that thousands of deaths from a virus even could be worthy of public concern. Sound familiar?
Reagan literally laughed in press conferences asking about citizens dying (video footage definitely not to be missed) as fatality numbers were read to him. The President also refused to let the national Center for Disease Control (CDC) communicate or be transparent about how to stop the spread.
Similarly, in the the current anti-science White House, a CDC response center was closed and communication shut down about viruses despite intelligence offices formally predicting a coming pandemic. In fact, the director of offices warning a virus would be a real national security concern was instead fired for fairly open political reasons.
It’s probably worth noting at this point that the current CDC director appointed in 2018 is infamous for his mismanagement and profiteering during the AIDS crisis, not to mention having no experience in directing a public health agency.
Redfield’s primary qualification for appointment seems to be his close association with extremist religious anti-science organizations. “Americans for a Sound AIDS/HIV Policy” (ASAP) spread propaganda that AIDS was “God’s judgment” against Americans who deserved to die because they were believed to be a result of single-parent households with weakened patriarchal values (weakened male domination over women).
A decision to slow down a SARS-CoV-2 testing in America may be related to Redfield looking for ways to corner and profit from test kit distribution channels, rather than jump right into big data acquisition/analysis or deploy tests kits ready to go (available from China since January 17th and openly distributed by WHO since February 6th, as exemplified by Singapore and Korea test data).
The real value, even to a corrupt huckster, should been seen as analytic platforms for data accumulation and subsequent analysis. That opportunity is many magnitudes greater than personal profit he may have salivated over when scheming about cornering test kit markets.
There’s no proof yet of this level of corruption causing the CDC test kit delays, it just seems incredibly likely given Redfield’s short-lived CDC predecessor Fitzgerald was forced to resign due to corruption, when it was reported she was investing in tobacco as grants went to a company where she and her husband held stock.
Perhaps it wasn’t corruption, though. It also could have been the kind of incompetence seen with CDC doing a huge drawdown in China, removing two-thirds of its experts in the past two years. Instead of long-term professional, localized scientific relationships designed to instantly collaborate on “the next SARS”, someone in America instead cooked up a concept of just-in-time teams for short-term pandemic expeditions.
The total and abject failure of this information gathering model was clear on January 29th when US officials personally pleaded with China to let their scientists back in.
…our hope is that we could get directly involved in China to be able to review…
On top of Redfield having learned the exact wrong lessons from America’s HIV response on the way to being appointed head of CDC during the current pandemic, there’s also the fact that Mike Pence was appointed to lead nationwide response despite his own infamous mismanagement of HIV.
In sum, America’s leadership “team have been dishonest about the coronavirus” spreading lies and sowing confusion just like Reagan did in the 1980s with AIDS, on top of enabling healthcare market fraud that inflates business profits while giving no coverage for scientific testing of coronavirus.
The lesson from the HIV crisis for current U.S. politicians therefore seems to have been the very opposite of preparation. There has been no hard drive to get a national command center for immediate pandemic test and containment, let alone any plans to update from Ebola-era mistakes to the latest and greatest big data technology (although they did just put out a feeler request for new investments).
Let’s be frank here, to some the only lesson of AIDS/HIV was… the American President can get away with indifference if not negligence, playing golf and refusing to lift a finger until it’s obvious why literally tens of thousands of Americans needlessly are dying on his watch.
BlueDot is notably Canadian.
So let’s go back to details of that BlueDot announcement for a minute. FP complained in 2014 that AI really meant just reading regular news channels and trying to take credit for it as novel. The core to that long quoted passage above, starting this blog post, is here:
…mainstream media still plays a critical role as an information stream in many areas of the world. This is not to say that there were not far earlier signals manifested in the myriad social conversations among medical workers and citizens in the region, only that it was not these indicators that HealthMap — or anyone else — detected…
That is quite literally what happened in China again this time. A detailed JAMA graph lays it out by day to clearly show a timeline of social conversations and then news stories. Click to enlarge.
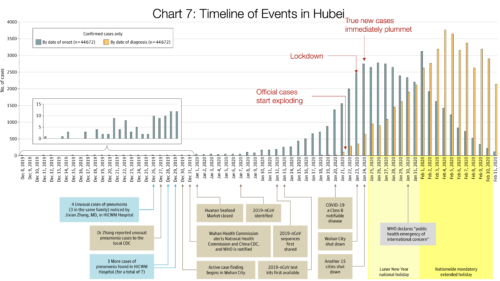
The text boxes are basically this:
- Dec 25: //not on JAMA timeline// The head of gastroenterology (Lu Xiaohong) at Wuhan City Hospital No. 5 says conversations were on disease spreading among medical workers treating a group of new pneumonia patients; several Chinese news outlets release reports from anonymous labtech claiming 87% similarity to SARS
- Dec 26: 4 unusual pneumonia cases noticed in HICWM Hospital by a Dr. Zhang
- Dec 27: Dr. Zhang reports unusual cases to government CDC (later review of records suggest as many 180 cases, probably not realized at this time)
- Dec 28-29: 3 more pneumonia cases in HICWM
- Dec 30: //not on JAMA timeline// Wuhan Central Hospital’s emergency department director (Ai Fen) uploads diagnostic record to WeChat, without knowing contagion rate
- Dec 30: government starts active case finding in Wuhan City
- Dec 30: //not on JAMA timeline// Wuhan Central Hospital ophthalmologist (Li Wenliang) mentions quarantined emergency patients to a WeChat group, indicating SARS-like virus
- Dec 30: //not on JAMA timeline// Program for Monitoring Emerging Diseases (ProMED) broadcasts English translation of Wuhan Municipal Health Committee RFI: “urgent notice on the treatment of pneumonia of unknown cause”
- Dec 31: Wuhan health officials formally release news to China’s national health officials including their CDC and the global WHO
And on Dec 30th BlueDot claimed credit for being the first to notice. This is quite exactly what FP was complaining about in 2014, when a machine reads the news and says it was early while being on the same timeline as an existing human global notification system.
To be fair, BlueDot was right there on the clock and neither claims AI to be a cure-all, nor that they were doing something amazing other than reading the news others were publishing. As they put it in their PR they “flagged articles in Chinese that reported 27 pneumonia cases associated with a market”.
Technically speaking that reference means BlueDot either read December 15th early reports about 27 cases (five days later the total number of confirmed cases had more than doubled to 60) or were repeating a Chinese government’s official December 30th announcement of 27 cases of viral pneumonia being investigated. It appears to be the latter.
While the system worked as designed, it still gets classified as a failure under the 2014 definition of high expectations for phrases like big data or AI. Local news and social channels reported the outbreak of pneumonia with SARS-like potential. Then people or machines both read that and flagged it as early warning signs of another SARS-like incident.
Reading newspapers around the world and reporting them on the same day was hot new technology of 1920. Hard to call this really newsworthy itself in 2020. As I said before, a lot has changed, while some has not. I wish BlueDot didn’t call their warnings early, and instead called them inexpensive or less complicated.
Nonetheless, if we allow the bar to be lowered to allow heavily funded startups to succeed and be measured for easier finish lines, BlueDot did indeed do what they advertised by reading news about SARS-like pneumonia as it was published and then repeating it for others to also read.
I’m not just pointing out a lowered bar has risk because I want to be captain obvious who says be wary of PR from startups. I actually believe we should hold the bar higher for them. There are technical solutions that really could give early warning signs that are ahead of the local reporters themselves, perhaps even before social conversations reach the reporters.
That is both why I’ve been writing my new book, and also is the focus of software I’m working on now. We can do better with big data technology, and we will.